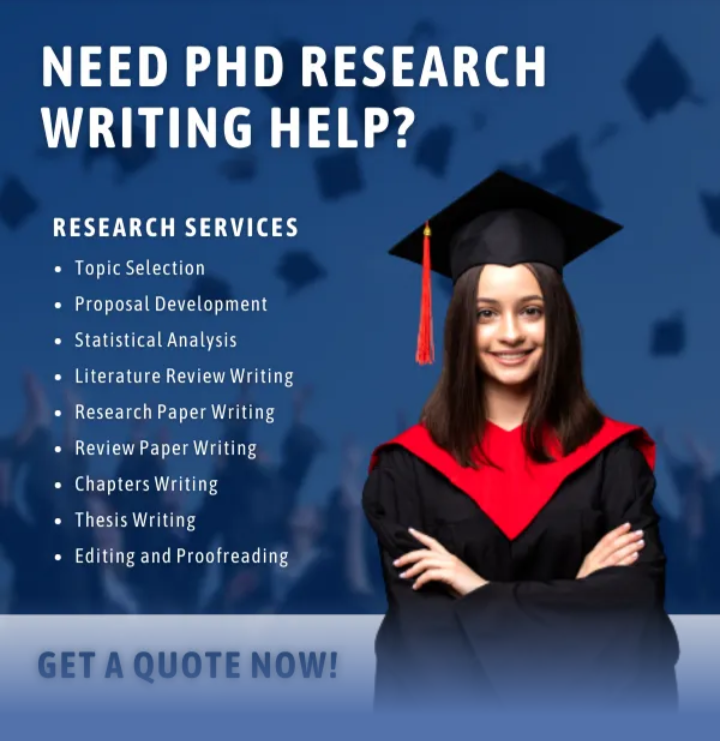
- Current Issue
- Past Issues
- Conference Proceedings
- Submit Manuscript
- Join Our Editorial Team
- Join as a Member
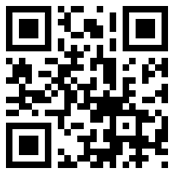
S.No | Particular | Page No. | |
---|---|---|---|
1 |
SHUBHI SAXENA Prof (Mr.) Mohd. ArifAbstract: |
![]() |
1-10 |
2 |
Sunita1, Dr. Vineeta Basotia2, Dr. Arun Kumar3Abstract: |
![]() |
11-18 |